Powerful statistical techniques can discern a desired audio signal from a jumble of inputs.
Dave Betts • AudioTelligence
Most of us live and work in the midst of noise. We have become used to contending with the sounds of other people talking, music playing, the TV blaring, traffic roaring… Our brains are pretty amazing at picking out the voice we want to hear amid the cacophony.
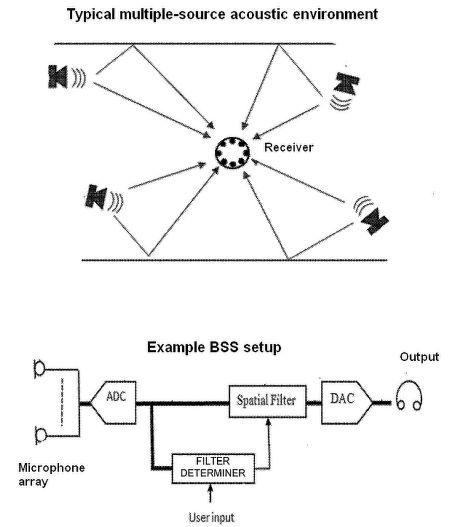
A typical acoustic scene that BSS addresses — four sources sending audio to an array of eight microphones. The aim of BSS is to de-mix the microphone signals to estimate the audio from the original sources with minimal information about the sources and locations of the microphones. Below, A typical scenario employing BSS to improve audibility of a source. A microphone array output is digitized by an analog/digital converter which provides multi-channel digitized audio to a spatial filter and to a filter coefficient determiner. The filter coefficient determiner calculates coefficients of a de-mixing filter which are applied by the spatial filter to extract audio from one or more of the microphones. The determiner optionally accepts user input to, for example, select one of the sources. De-mixed audio then goes to a digital/analog converter and then to loudspeakers.
But, for anyone with mild to moderate hearing loss, it can be difficult to hear one voice clearly against all the background noise. It is a problem that affects an estimated 80% of us as we reach middle age.
Electronic devices have the same issue — the audio signals picked up by their microphones are often contaminated with interference, noise and reverberation. The quality and intelligibility of the speech which the devices are designed to capture can be badly affected.
Signal processing techniques, such as beamforming and blind source separation (BSS), can come to the rescue. But which should you choose for which audio application – and why?
Beamforming
Audio beamforming is one of the most versatile multi-microphone methods for emphasizing a particular source in an acoustic scene. In its simplest form — called a delay-and-sum beamformer — the microphone signals are delayed (usually digitally) to compensate for the different path lengths between a target source and the different microphones. The beamformer reinforces the sound signals coming from a particular direction.
However, sound does not move in straight lines: A given source has multiple different paths to the microphones, each with differing amounts of reflection and diffraction. So a simple delay-and-sum beamformer is not very effective at extracting a source of interest from an acoustic scene. But it is very easy to implement and does give a small amount of benefit, so it was often used in older devices.
Many more advanced beamforming techniques are now available. However, to extract the source of interest, they all require information about the direction of the sources and the locations of the microphones. In more detail the input signals are usually split into frequency bands and each frequency band is treated independently, and then the results at different frequencies are recombined. Some such techniques also can be sensitive to the accuracy of microphone and source location and may reject the target source because it does not actually come from the indicated direction.
More modern devices often use adaptive sidelobe cancellation, which tries to cancel out the sources that are not from the direction of interest. These are state-of-the-art in modern hearing aids, for example, and allow the user to concentrate on sources directly in front of them. But the significant drawback is that you have to be looking at whatever you are listening to, and that may be awkward if your visual attention is needed elsewhere — for example, when you are grabbing a cup of coffee while talking to someone.
So beamforming is helpful if you need a method of enhancing audio which is low cost and reliable, provided your use case only requires a small amount of improvement — say, around 2dB. It can also work well in large, fixed microphone arrays for meeting rooms and conference centers, where the microphones together focus a narrow beam on a small area — for example, on the speaker at a podium.
Blind source separation
BSS is a family of powerful techniques that separate the acoustic scene into its constituent parts by making use of statistical models of how sources generally behave. BSS extracts all the sources, not just one, and does so without any prior knowledge of the sources, the microphone array or the acoustic scene.
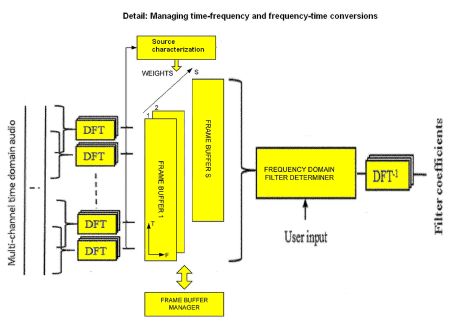
A more detailed view of BSS conversion between time-frequency and vice versa as happens in the frequency domain filter coefficient determiner. Each audio channel has a short time Fourier transform (STFT) module configured to perform a succession of overlapping discrete Fourier transforms (DFTs) on an audio channel, generating a time sequence of spectra. Transformation of filter coefficients back into the time domain happen via inverse DFTs.
BSS works from the audio data rather than the microphone geometry. This makes it insensitive to calibration issues and generally able to achieve much higher separation of sources in real-world situations than any beamformer. And, because it separates all the sources irrespective of direction, it can follow a multi-way conversation automatically. This is particularly helpful for hearing assistance applications, where the user wishes to follow a conversation without having to interact with the device manually. BSS can also be effective when used in home smart devices and in-car infotainment applications.
Generally speaking, BSS separates acoustic sources by first taking snapshots of acoustic data in a time-frequency domain. A multichannel filter operates on these data frames to separate signals from acoustic sources. Moreover, the filter is reconfigured on the fly. The procedure involves determining a set of what are called de-mixing matrices that are applied to each data frame to determine changes in the separated outputs. Each de-mixing matrix is modified for each detected frequency depending on trends in the acoustic data.
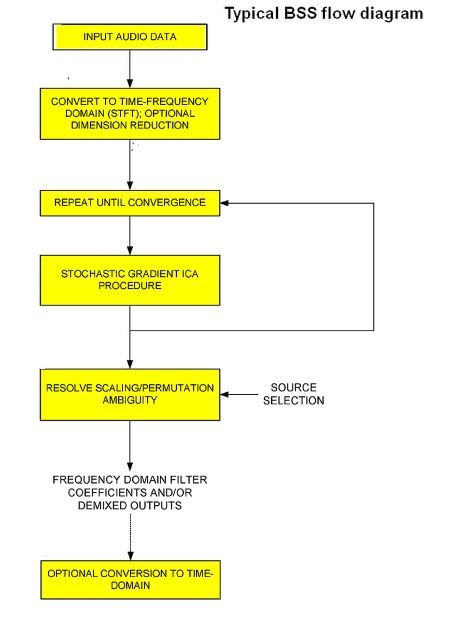
A typical flow diagram for BSS. Audio data is converted to the time-frequency domain, optionally reducing the number of audio channels. Then an importance weighting/trend detection procedure repeats until it converges. This hopefully resolves scaling and ambiguities in the signal, at which point filter coefficients get converted back to the time domain.
However, BSS is not without its own problems. For most BSS algorithms, the number of sources that can be separated depends upon the number of microphones in the array. And, because it works from the data, it needs a consistent frame of reference, which currently limits the technique to devices which have a stationary microphone array — for example, a table-top hearing device or a microphone array for fixed conferencing systems.
When there is background babble, BSS will generally separate the most dominant sources in the mix, which may include the annoyingly loud person on the next table. So, to work effectively, BSS needs to be combined with an ancillary algorithm for determining which of the sources are the sources of interest – but dynamically following those sources in a conversation is easy.
There is also the issue of latency if BSS is used for hearing devices. These need ultra-low latency to keep lip sync, which is a difficult problem to solve. BSS algorithms with only 5 msec latency have only recently been developed — after years of research.
Whether you choose BSS or beamforming to enhance audio depends on your needs. If you can accept a limited improvement in the sound, and cost is a major consideration, beamforming is relatively cheap and easy to implement. But if your use case requires the separation of different signals and a large improvement in the signal-to-noise ratio — or ultra-low latency for hearing solutions — BSS may be the answer. DW
You may also like:
Filed Under: Medical-device manufacture, MOTION CONTROL, ELECTRONICS • ELECTRICAL